FAIR Workshop on Digital Trace Data in Social Science Research
The interdisciplinary research area FAIR organizes a three-day workshop on Digital Trace Data in Social Science Research.
In this workshop, participants will be introduced to digital trace data and its collection (block 1) as well as the analysis of digital trace data, especially text data (block 2).
Our invited speakers are:
- Prof. Dr. Frauke Kreuter a,b
- Dr. Anna-Carolina Haensch a
- Prof. Dr. Christoph Kern a,c
- Clara Strasser Ceballos a
a Ludwig-Maximilians-Universität München
b University of Maryland
c Mannheim Centre for European Social Research
When and where
Dates and Time:
June 26, 2024, 10:00 - 17:00 CET
June 27, 2024, 10:00 - 17:00 CET
June 28, 2024, 10:00 - 17:00 CET
Location: Essen (on-site)
How to register
To register for the workshop, please send an email to workshop.fairtu-dortmundde . Please provide your name, institution (e.g. TU Dortmund), faculty (e.g. Faculty of Social Science), status (e.g., doctoral researcher, Postdoc) and a short overview of your experience and your goals regarding digital trace data in social science research (max. 100 words).
The deadline for registration is 31st May 2024.
Invited Speakers:
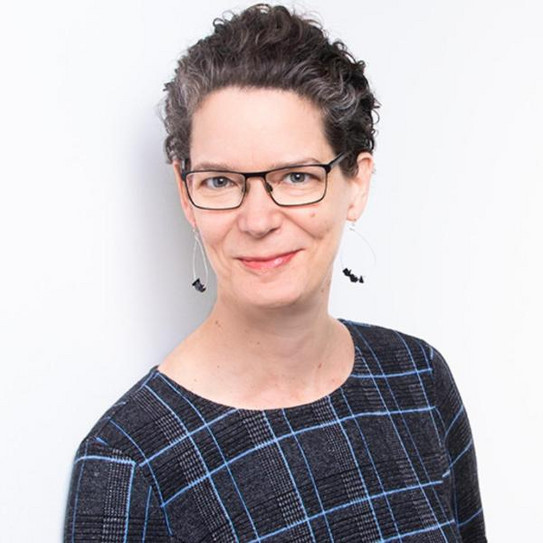
Frauke Kreuter
Frauke Kreuter bridges people, challenges, and organizations, to enhance data quality not only for AI models.
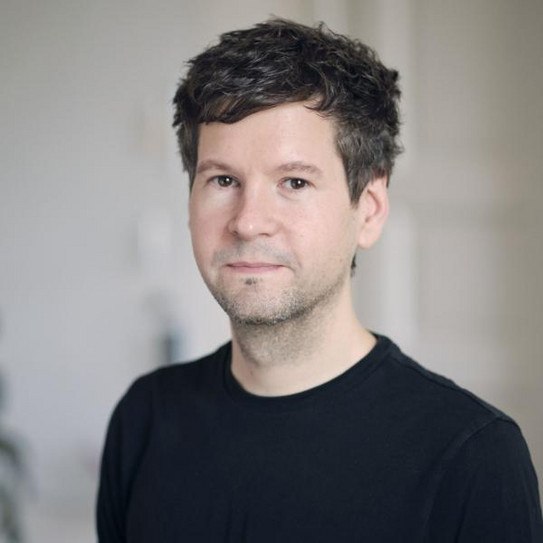
Christoph Kern
Christoph Kern's research focuses on the social impacts of algorithmic decision-making and on methodology to mitigate algorithmic unfairness and improve training data quality.
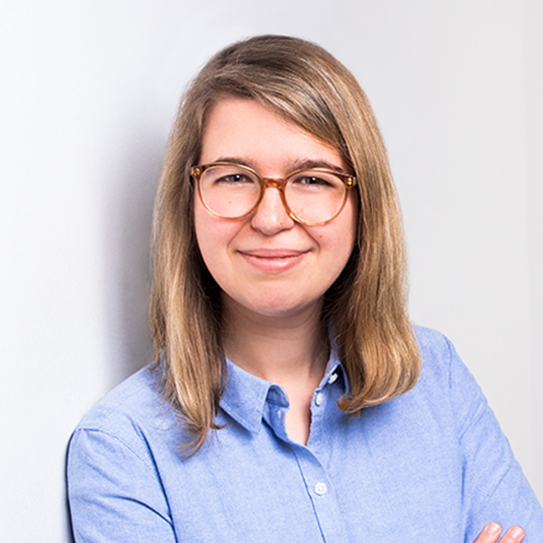
Caro Haensch
Caro Haensch is exploring the new frontiers of social data science by merging her survey statistics background with a pioneering approach to synthetic data and large language models.
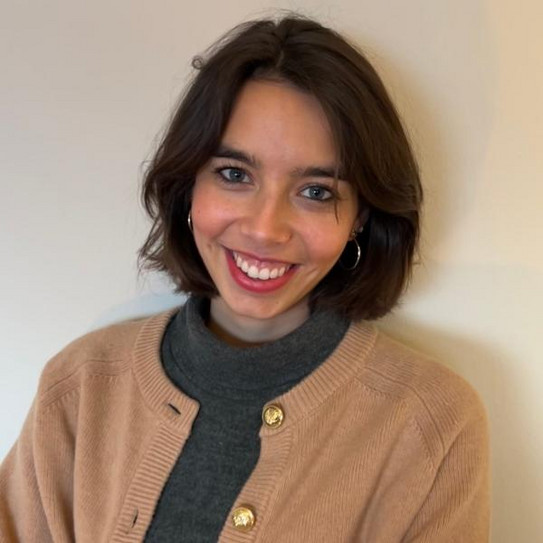
Clara Strasser
Clara Strasser Ceballos harnesses the power of data for the social good, by shaping social-aware and fair AI systems in her interdisciplinary research.
The workshop, "Digital Trace Data in Social Science Research" is structured into two main sections:
- Overview of Digital Trace Data: This section introduces digital trace data, exploring various sources like e-learning systems, websites, smartphone apps, and sensors in wearables. Key aspects covered include the typical characteristics of these data, data quality, and their potential for social and cultural science research, along with the prerequisites for leveraging these potentials. Special attention is given to social media data from platforms like YouTube, Reddit, and TikTok. The session includes both theoretical discussion and practical data collection exercises using the statistical programming language R.
- Analysis of Digital Trace Data: The second section delves into the analysis of the data discussed earlier. It begins with an introduction to supervised and unsupervised machine learning, covering use cases and methods. It then focuses on specific applications: text classification models (an example of supervised learning) and topic modeling (an example of unsupervised learning). Participants will engage in practical R exercises to consolidate their learning. The workshop concludes with a forward-looking segment that explores the application of these methods to other data formats, such as analyzing open-ended responses in traditional survey data.
Outline:
- Social media data (Caro Haensch): Overview of data sources and data collection
- General insight into digital trace data (Frauke Kreuter, online via Zoom): Data quality
- Data collection (Caro Haensch & Clara Strasser Ceballos): Hands-on data collection through Scraping and APIs
- Analysis (Christoph Kern & Clara Strasser Ceballos): Overview: supervised and unsupervised machine learning, Classification models for text (Supervised ML), Topic modeling (unsupervised ML)
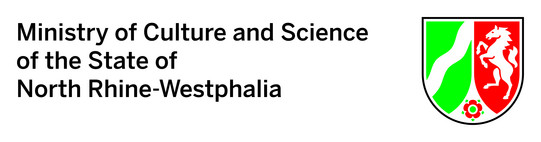
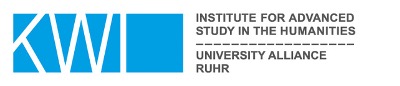