FAIR Graduate programm in statistical methods - Summer semester 2023
The interdisciplinary research area FAIR will offer a graduate program in statistical methods starting in the summer semester 2023. This program is primarily for doctoral students in the social sciences, but also post-docs and doctoral students of other sciences are welcome. The lectures will take place during the summer break in August and September, once a week. The lectures are (mostly) independent from each other, thus a partial participation is also possible. Each session will contain a practical "hands-on" part, in R programming language. The language of the lectures and of the accompanying materials is English and German.
How to register?
There is a Moodle space for the registration, as well for the (future) access to the learning materials.
Schedule
The course will take place in person at the TU. The lecture room will be M/E27 in Mathetower.
The tentative schedule is (the final schedule will be confirmed later):
- 03.08.2023 14:00-16:00 and 16:00-18:00 — A. Krivošija: Time Series and Geometric Learning
- 17.08.2023 14:00-16:00 and 16:00-18:00 — P. Doebler: Lasso regularization
- 31.08.2023 14:00-16:00 and 16:00-18:00 — S. Frick: Item Response Theory
- 07.09.2023 14:00-16:00 and 16:00-18:00 — B. Zeyer-Gliozzo: Panel Data
- 21.09.2023 14:00-16:00 and 16:00-18:00 — J. Schwerter: Differences in Differences
- 28.09.2023 14:00-16:00 and 16:00-18:00 — L. Amro: Bootstrapping
Abstracts
Title: Supervised Machine Learning for Social and Behavioral Scientists: Lasso regularization
Lecturer: Prof. Dr. Philipp Doebler
The short course focuses on an introduction to supervised learning models and their application in social and behavioral science. Learning goals include (i) understanding core concepts of supervised machine learning and their implication for data analysis, (ii) advantages and limitations of regularized regression models for low, medium and high dimensional data with a focus on the Lasso, and (iii) practical hands-on knowledge of related software.
Title: How to Deal with Large Time Series?
Lecturer: Dr. Amer Krivošija
The goal of this course is to introduce the various dissimilarity measures of time-series (and not only well-known Dynamic Time Warping), from the point of view of a theoretical computer scientist, and how these can be adapted to various applications. The techniques for simplification and sketching - make it simpler to handle noise and/or overfitting. We discuss some realistic assumptions - and (why) do we need them?
Title: Introduction to Item Response Theory
Lecturer: Dr. Susanne Frick
The short course introduces the basics of item response theory and its use in analyzing and constructing tests. Learning goals include (i) understanding core concepts of IRT, (ii) assessing fit and differential item functioning, (iii) practical hands-on knowledge of related software.
Title: Fixed-Effects-, Random-Effects-, and “Hybrid” Models for Panel Data
Lecturer: Birgit Zeyer-Gliozzo
The short course focuses on the analysis of panel data (i.e., data on the same unit, such as individuals or households, over multiple points in time), contrasting different methods with examples of applications: Pooled OLS, Random-Effects- (RE) Models, Fixed-Effects- (FE) Models, and “Hybrid” Models, which combine desirable features of the last two methods. Learning objectives include (i) understanding the core concepts of the different methods, (ii) knowledge of the advantages and limitations of each method, and (iii) practical hands-on knowledge of the corresponding software.
Title: Permutation and Bootstrap Procedures for Testing Hypotheses
Lecturer: Dr. Lubna Amro
The short course focuses on permutation and bootstrap procedures for inference, specifically in the context of hypothesis testing. Learning outcomes include (i) understanding core concepts of the process of hypothesis testing, bootstrapping, and permutation testing, (ii) knowledge of the benefits of permutation and bootstrap procedures over traditionally used parametric tests, (iii) working with count, ordinal, or even ordered categorical data, and techniques for working with repeated measures data, and (iv) practical hands-on knowledge of related software.
Title: Introduction to non-experimental causal methods with emphasis on difference-in-differences
Lecturer: Dr. Jakob Schwerter
The short course focuses on the question of how to estimate a causal effect in a situation in which a random-control trial study was not possible to conduct. Therefore, a short introduction into common non-experimental methods will be given with an emphasis on difference-in-differences and its current developments. Learning goals include (i) understanding core concepts of causal inference with an emphasize on difference-in-differences and its implication for data analysis, (ii) limitations of the two-way fixed-effects estimation and possible solutions, and (iii) practical hands-on knowledge of related software.
Lecturers
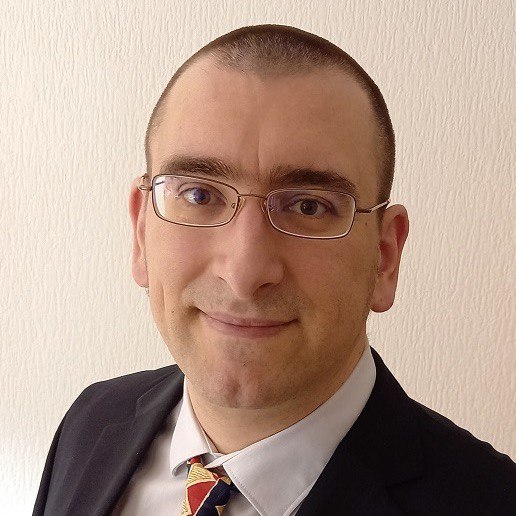
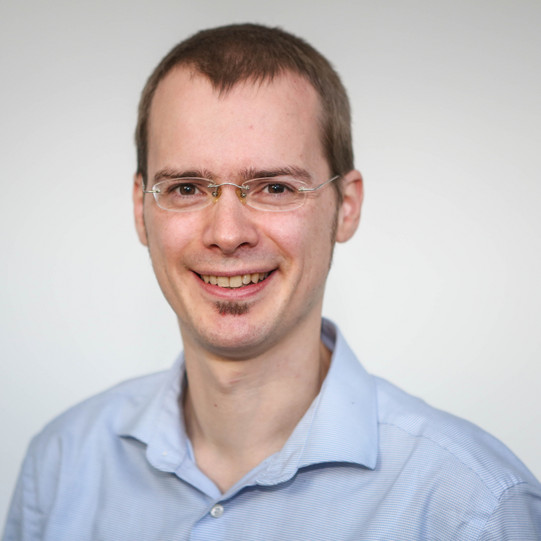
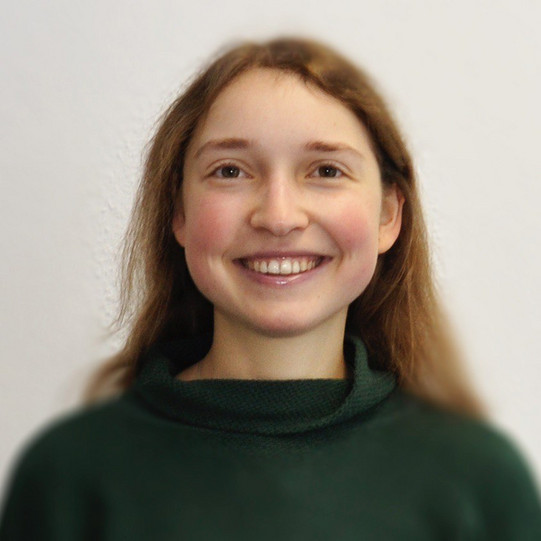
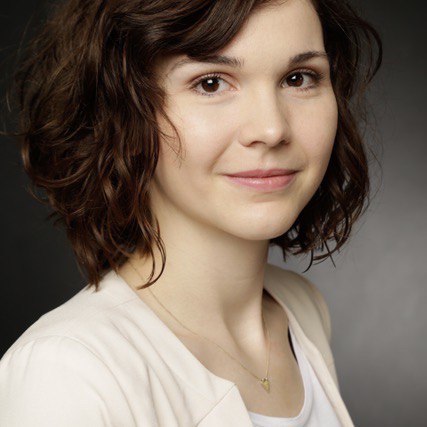
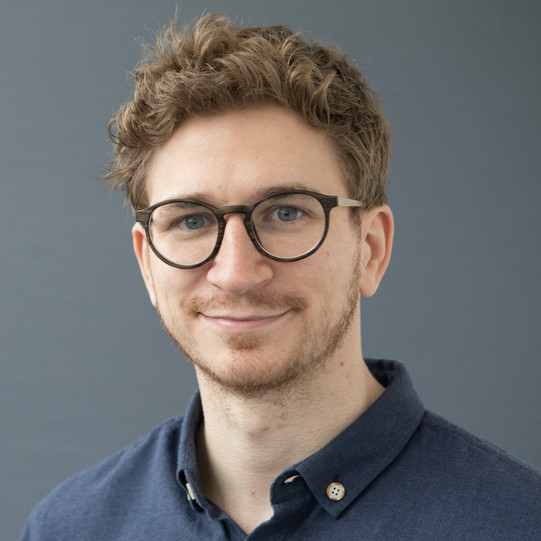
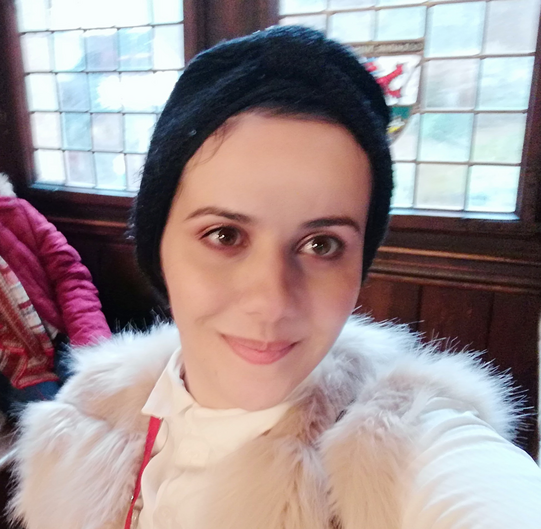
For inquiries please write an email to Amer Krivošija (amer.krivosijatu-dortmundde).