FAIR Graduate programm in statistical methods - Summer semester 2024
The interdisciplinary research area FAIR will offer a graduate program in statistical methods again in the summer semester 2024. This program is primarily for doctoral students in the social sciences, but also post-docs and doctoral students of other sciences are welcome. The lectures will take place during the summer break in August and September, once a week. The lectures are (mostly) independent from each other, thus a partial participation is also possible. Each session will contain a practical "hands-on" part, in R programming language. The language of the lectures and of the accompanying materials is English and German (it will be specified for each lecture separately).
How to register?
There is a Moodle space for the registration, as well for the (future) access to the learning materials.
Schedule
The course will take place in person at the TU. The lecture room will be M/E27 in Mathetower.
The tentative schedule is (the final schedule will be confirmed later):
- 01.08.2024. 14:00-18:00 — P. Doebler: Lasso regularization and random forest
- 08.08.2024. 14:00-18:00 — E. Graf: Causal inference with observational data
- 15.08.2024. 14:00-18:00 — J. Schwerter: Introduction to Causal Machine Learning
- 22.08.2024. 14:00-18:00 — S. Frick: Item Response Theory
- 29.08.2024. 14:00-18:00 — F. Krieger: Latent Growth Curve Models
- 05.09.2024. 14:00-18:00 — B. Zeyer-Gliozzo: Panel Data
- 12.09.2024. 14:00-18:00 — L. Amro: Bootstrapping
- 19.09.2024. free
- 26.09.2024. 14:00-18:00 — A. Krivošija: On Clustering and Trajectories
Abstracts
Title: Supervised Machine Learning for Social and Behavioral Scientists: Lasso regularization and random forest
Lecturer: Prof. Dr. Philipp Doebler
The short course focuses on an introduction to supervised learning models and their application in social and behavioral science. Learning goals include (i) understanding core concepts of supervised machine learning and their implication for data analysis, (ii) advantages and limitations of regularized regression models for low, medium and high dimensional data with a focus on the Lasso, (iii) a gentle introduction to tree-based learning models towards useful working knowledge of the random forest, and (iv) practical hands-on knowledge of related software.
This course will provide you the basics which might help for the course on causal machine learning, in case you do not have prior knowledge or would wish a recap on machine learning.
Title: Introduction to causal inference with observational data
Lecturer: Dr. Elisabeth Graf
The short course focuses on the question of how to estimate a causal effect when a randomized controlled trial is not feasible. The course will provide a short introduction into the potential outcome framework and common non-experimental methods (e.g., regression discontinuity design or difference-in-differences). Learning goals include (i) understanding core concepts of causal inference and its implication for data analysis, (ii) identifying advantages and limitations in the application, and (iii) gaining practical hands-on knowledge of related software.
This course will provide you the basics which might help for the course on causal machine learning, in case you do not have prior knowledge or would wish a recap on machine learning.
Title: Introduction to Causal Machine Learning
Lecturer: Dr. Jakob Schwerter
The short course builds on the short courses on Causal Inference and Machine Learning and shows how and in which settings machine learning can be used to answer causal research questions. Learning goals include (i) understanding core concepts of causal machine learning and their implications for data analysis, (ii) advantages and limitations of causal machine learning, and (iii) practical hands-on knowledge of related packages in R.
Title: Introduction to Item Response Theory
Lecturer: Dr. Susanne Frick
The short course introduces the basics of item response theory and its use in analyzing and constructing tests. Learning goals include (i) understanding core concepts of IRT, (ii) assessing fit and differential item functioning, (iii) practical hands-on knowledge of related software.
Title: Latent Growth Curve Models
Lecturer: Dr. Florian Krieger
This short course provides a practical introduction to Latent Growth Curve Models (LGCM), which are ideal for the analysis of longitudinal data, e.g. for modeling learning trajectories. This short course is aimed at participants who are interested in a practical introduction to latent growth models and want to learn how to apply them to real data sets. At the end of the short course, participants will be able to specify and interpret latent growth curve models, graphically represent the results and present them in scientific papers.
Title: Fixed-Effects-, Random-Effects-, and “Hybrid” Models for Panel Data
Lecturer: Dr. Birgit Zeyer-Gliozzo
The short course focuses on the analysis of panel data (i.e., data on the same unit, such as individuals or households, over multiple points in time), contrasting different methods with examples of applications: Pooled OLS, Random-Effects- (RE) Models, Fixed-Effects- (FE) Models, and “Hybrid” Models, which combine desirable features of the last two methods. Learning objectives include (i) understanding the core concepts of the different methods, (ii) knowledge of the advantages and limitations of each method, and (iii) practical hands-on knowledge of the corresponding software.
Title: Permutation and Bootstrap Procedures for Testing Hypotheses
Lecturer: Dr. Lubna Amro
The short course focuses on permutation and bootstrap procedures for inference, specifically in the context of hypothesis testing. Learning outcomes include (i) understanding core concepts of the process of hypothesis testing, bootstrapping, and permutation testing, (ii) knowledge of the benefits of permutation and bootstrap procedures over traditionally used parametric tests, (iii) working with count, ordinal, or even ordered categorical data, and techniques for working with repeated measures data, and (iv) practical hands-on knowledge of related software.
Title: On Clustering and Trajectories
Lecturer: Dr. Amer Krivošija
The goal of this course is to give an overview of different clustering approaches - hierarchical and center-based. The importance of the distance measure used for clustering is shown. In particular this choice is important for clustering of trajectories (time series, polygonal curves, panel data, longitudinal data), since they contain time stamps as an additional information that should not be discarded.
Lecturers
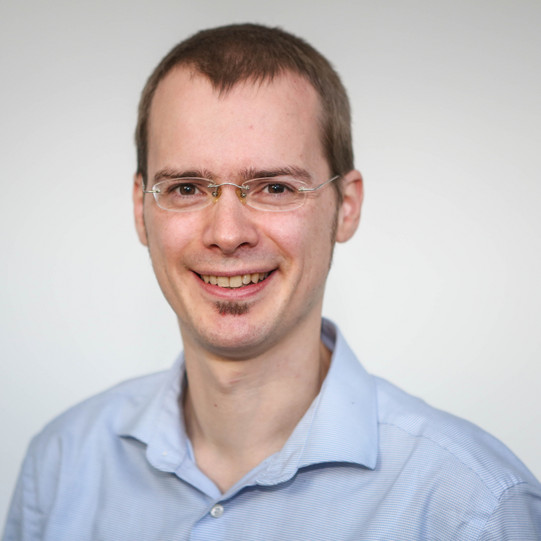
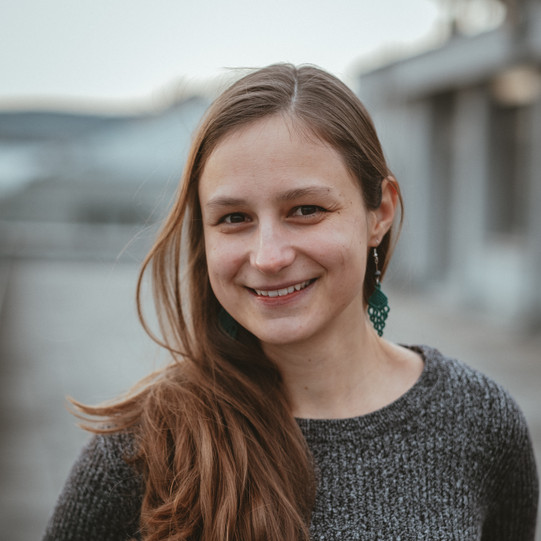
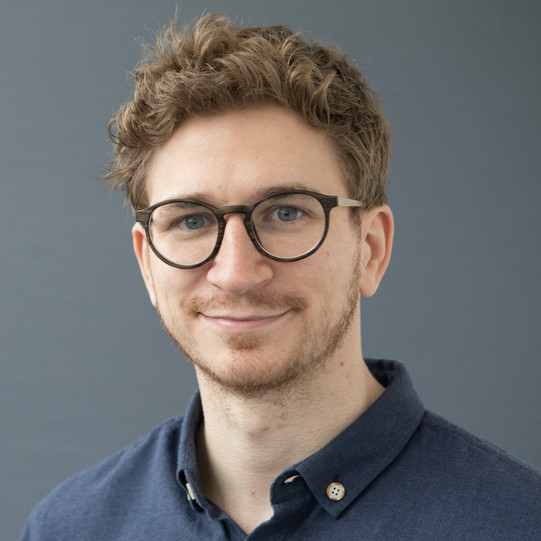
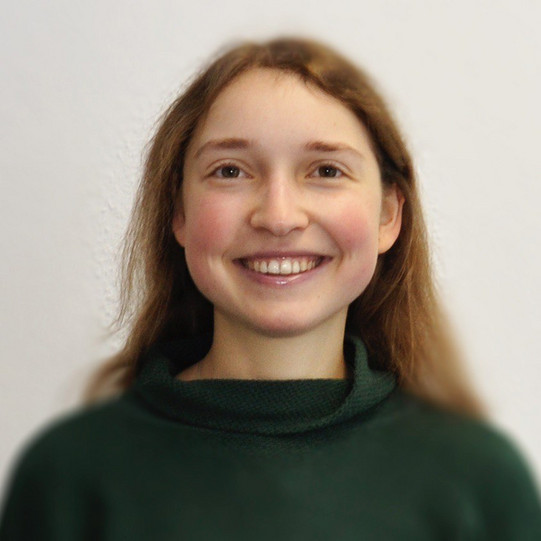
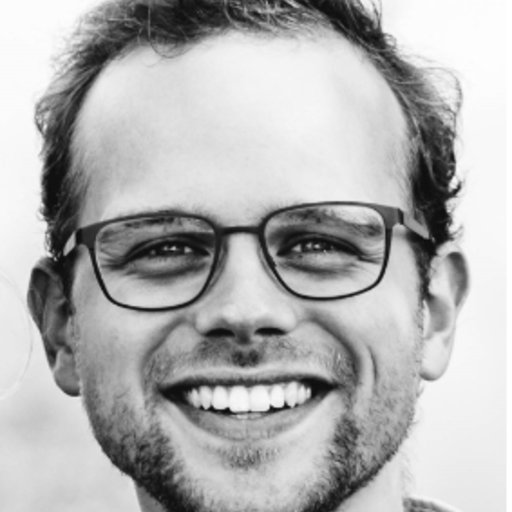
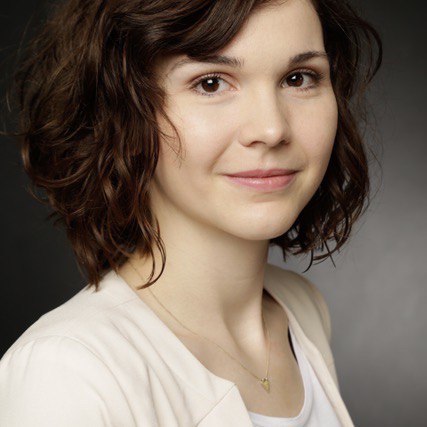
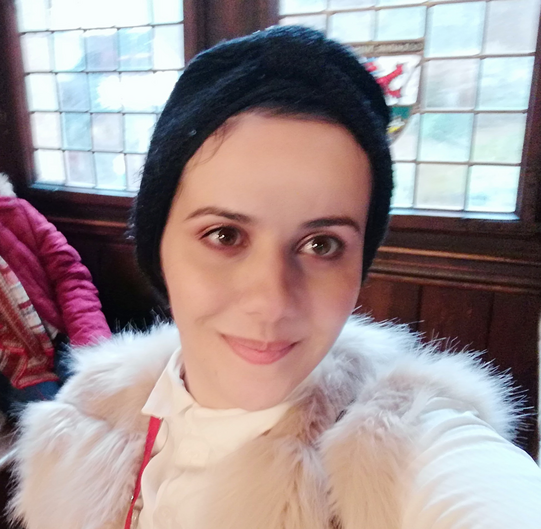
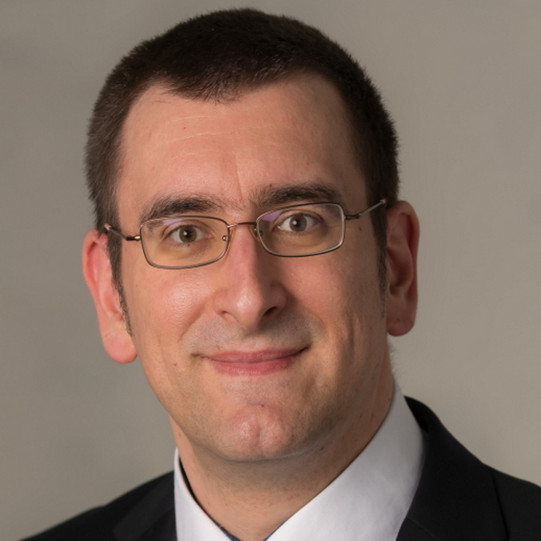
For inquiries please write an email to Amer Krivošija (amer.krivosijatu-dortmundde).