FAIR Workshop on Using Resampling and Simulation to Tackle Heterogeneity in Social Science Research
The interdisciplinary research area FAIR organizes a two-day workshop on Using Resampling and Simulation to Tackle Heterogeneity in Social Science Research "in cooperation with the Research Center Trustworthy Data Science and Security".
This workshop will introduce participants to a range of topics, including simulation techniques, resampling procedures, multivariate data analysis, regression analysis, handling missing values, and imputation techniques.
Our invited speakers are:
- Moritz Berger (University of Bonn)
- Dennis Dobler (Vrije Universiteit Amsterdam)
- Florian Dumpert (Federal Statistical Office of Germany)
- Sarah Friedrich (University of Augsburg)
- Frank Konietschke (Charité – Universitätsmedizin Berlin)
- Łukasz Smaga (Adam Mickiewicz University)
When and where
Date and Time: September 22 and 25, 2023. 9:00-14:30 CET
Location: TU Dortmund University, room: Otto-Hahn-Strasse 12, E.003
Virtual: Online in Zoom (a link will be sent only to registered participants by email)
How to register
Registration is necessary both for on-site and online participation. In order to register for this workshop, please fill out the following online form: https://umfragen.tu-dortmund.de/index.php/386944?lang=en
The extended deadline for registration is 19th September 2023.
Invited Speakers
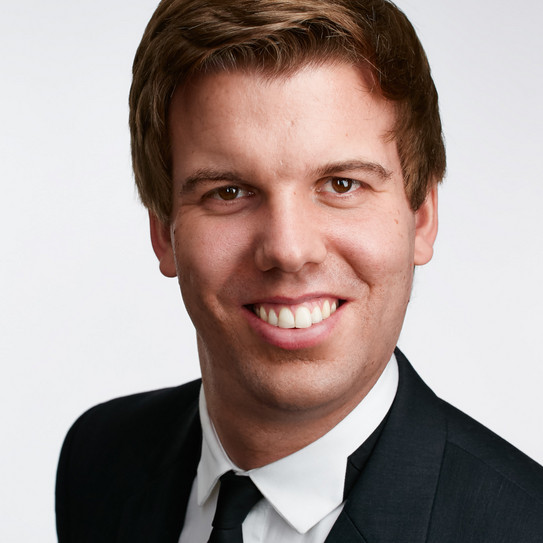
Moritz Berger
University lecturer at Institute of Medical Biometry, Informatics and Epidemiology (IMBIE), Faculty of Medicine, University of Bonn
Moritz obtained his PhD at LMU Munich under the supervision of Gerhard Tutz. Subsequently, he joined the IMBIE at University of Bonn as Postdoc. In 2022 he completed his Habilitation in Medical Biometry. Moritz’ research interests include regularization and variable selection, time-to-event analysis, tree-based approaches as well as categorical data analysis.
Website: https://www.imbie.uni-bonn.de/people/dr-moritz-berger/
Parametric and Nonparametric Modeling of Dispersion Effects in Ordinal Regression
Abstract: The proportional odds model is probably the most widely applied ordinal regression model. It postulates that the effects of the explanatory variables on the outcome are the same across all categories. This facilitates the interpretation of model parameters in terms of cumulative odds, which makes it very attractive for practitioners. In many applications, however, the assumptions of the proportional odds model are too restrictive. In particular, if the outcome shows differing variability in subgroups of the population (also referred to as dispersion) the proportional odds model will typically show poor goodness-of-fit. An extended model that accounts for heterogeneity of variances is the location-scale model already considered by McCullagh (1980) also known as heteroscedastic logit model. More recently, we proposed the so-called location-shift model as an alternative to account for varying dispersion. The location-shift model contains the familiar location term (as in the proportional odds model) complemented by a linear term that represents variability of the outcome. Parameters of the location-shift model can be interpreted straightforward in terms of log odd ratios. Moreover, it can be embedded into the framework of multivariate generalized linear models.
In this talk, by several real-world applications (e.g. data of the general social survey of social science; ALLBUS) we demonstrate that the location-shift model frequently shows satisfactory goodness-of-fit while being comparably sparse in parameters. In addition, we introduce the class of additive location-shift models allowing for smooth effects, as well as a tree-structured extension of the location-scale model accounting for interactions between the explanatory variables.
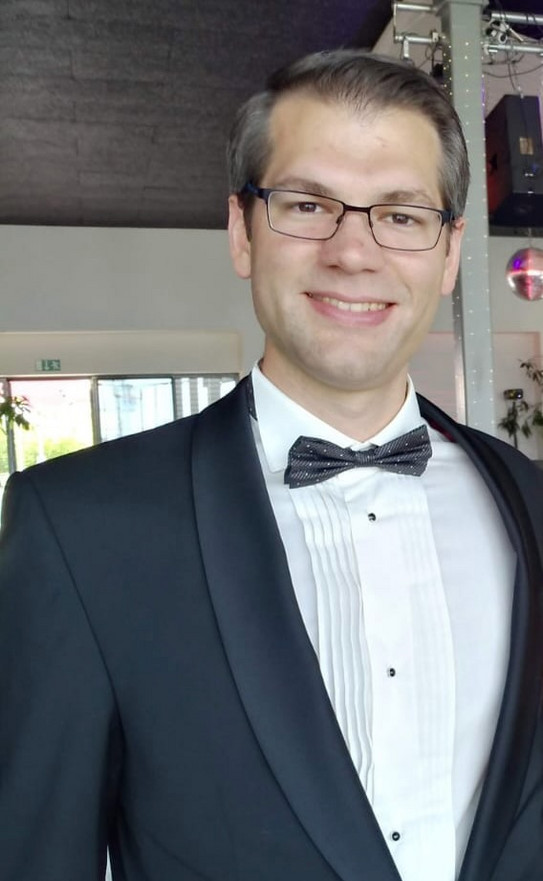
Dennis Dobler
Assistant Professor for Mathematical Statistics at the Department of Mathematics of Vrije Universiteit Amsterdam
After studying mathematics at Heinrich-Heine-University Düsseldorf, Dennis obtained his PhD under the supervision of Markus Pauly in 2016 at Ulm University. In 2017, he was appointed as tenure track assistant professor at Vrije University Amsterdam.
Dennis' research interests include survival analysis, resampling methods, missing data, multivariate analysis, non- and semiparametric statistics, machine learning algorithms.
Website: http://www.few.vu.nl/~ddr740/
Randomization-based Inferece in Nonparametric Repeated Measure Models with Missing Data
Abstract: Relative effects enjoy great popularity across various field of research. Also in statistical methodolgy research, extensions of this method have been developed in many different directions. In this talk, we will focus on repeated measures designs with randomly missing data. Here, relative effects can be used to find a time or other effects on the outcomes. In a previous SMMR-paper by Rubart, Pauly, and Konietschke (2022), relevant theory has been developed for tests based on quadratic forms in this context. They developed Wald- and ANOVA-type tests that are based on approximations using estimated chi-squared and F-distributions. In this talk, we re-visit that testing problem by means of a randomization procedure which will give rise to asymptotically exact inference procedures. Simulations demonstrate the small sample performance and a real data analysis illustrates several aspects of our method.
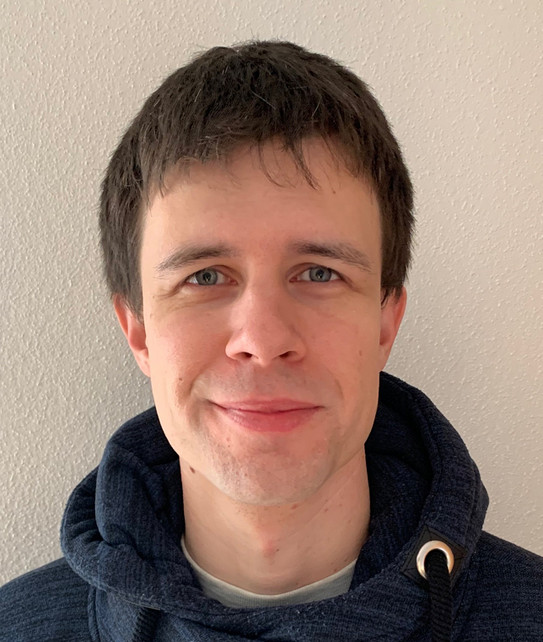
Florian Dumpert
Affiliation: Section for Artificial Intelligence and Big Data of the Federal Statistical Office of Germany (Destatis)
Short CV: Advancing official statistics through a quality-assured introduction of machine learning in the statistical production process is an important concern of Florian. For about ten years, the mathematician has been dealing with questions of statistical machine learning in science and administration. At the Federal Statistical Office of Germany, he now works with the unit responsible for artificial intelligence, machine learning, data-driven editing, imputation and Big Data techniques.
Classify and code, review and validate, and edit and impute in German official statistics
Abstract: Official statistics face a variety of challenges. Driven by the increased possibilities of obtaining information and the progress in information technology, the demand for information from politics, the economy and society on the most diverse subject areas of official statistics is increasing. In order to meet this demand adequately, the production of statistics must be further developed. This is not only a matter of making new data sources usable, but also of making processes more efficient. This applies in particular to steps in the area of data processing, such as classify and code, review and validate, and edit and impute. Solutions for (partial) automation of the processing steps are therefore being tested and used by Destatis.
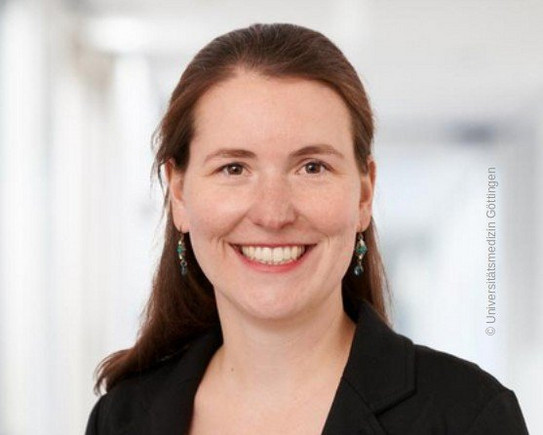
Sarah Friedrich
Sarah obtained her PhD at the Institute of Statistics, Ulm University, under the supervision of Markus Pauly. After spending a year as a post-doc in Copenhagen, she became a junior professor for Computational Statistics at the University Medical Center Göttingen. Since 2021, she is professor for Mathematical Statistics and Artificial Intelligence in Medicine at the Institute of Mathematics, University of Augsburg.
Website: https://www.uni-augsburg.de/de/fakultaet/mntf/math/prof/msaim/arbeitsgruppe/friedrich/
On the role of benchmarking data sets and simulations in method comparison studies
Abstract: Method comparisons are essential to provide recommendations and guidance for applied researchers, who often have to choose from a plethora of available approaches. While many comparisons exist in the literature, these are often not neutral but favor a novel method. Apart from the choice of design and a proper reporting of the findings, there are different approaches concerning the underlying data for such method comparison studies. Most manuscripts on statistical methodology rely on simulation studies and provide a single real-world data set as an example to motivate and illustrate the methodology investigated. In the context of supervised learning, in contrast, methods are often evaluated using so-called benchmarking data sets, that is, real-world data that serve as gold standard in the community. Simulation studies, on the other hand, are much less common in this context. In this talk, we will investigate differences and similarities between these approaches, discuss their advantages and disadvantages, and ultimately develop new approaches picking the best of both worlds.
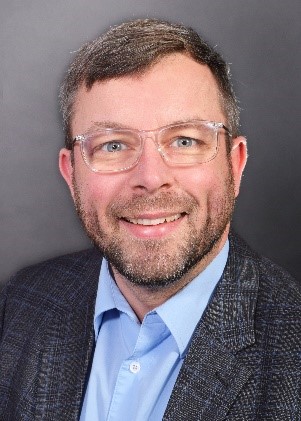
Frank Konietschke
Frank obtained his PhD in Mathematics from University of Göttingen. He now is full professor of Biostatistics and director of the Institute of Biometry and Clinical Epidemiology at the Charité Universitätsmedizin Berlin. His research primarily focuses on small sample size inference in various designs and methods, including resampling methods, nonparametric statistics, high-dimensional data analysis as well as diagnostic trial. He further is deputy head of the working groups on non-clinical statistics of the International Biometric Society (German Region).
Website: https://biometrie.charite.de/metas/person/person/address_detail/prof_dr_frank_konietschke/
Max – t Test in high dimensional Repeated Measures Designs
Abstract: In many experiments and especially in translational and preclinical research, sample sizes are (very) small. In addition, data designs are often high dimensional, i.e. more dependent than independent replications of the trial are observed. In this talk, we discuss the use of max t-tests (also known as multiple contrast tests) in high dimensional repeated measures and multivariate designs. We hereby relax the usual but rather strict assumptions of multivariate normality and/or equal covariance matrices across the different (independent) samples. We derive the limiting distribution of the test statistics and propose a wild bootstrap approach as approximate solution for small sample sizes. Extensive simulation studies indicate that the test controls the type-1 error very well even when sample sizes are as small as 10. A real data set illustrates the application of the methods.
References:
Konietschke, F., Schwab, K, Pauly, M. (2020). Small sample sizes: A big data problem in high-dimensional data analysis. Statistical Methods in Medical Research DOI: 10.1177/0962280220970228
Lin, Z., Lopes, M. E., & Müller, H. G. (2023). High-dimensional MANOVA via bootstrapping and its application to functional and sparse count data. Journal of the American Statistical Association, 118(541), 177-191.
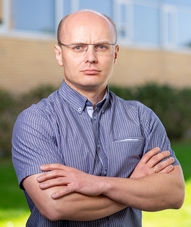
Łukasz Smaga
Associate professor at the Department of Mathematical Statistics and Data Analysis at the Faculty of Mathematics and Computer Science of the Adam Mickiewicz University in Poznań
Łukasz obtained his Ph.D. and habilitation (D.Sc.) at the Faculty of Mathematics and Computer of the Adam Mickiewicz University in Poznań. He now works there in the position of associate professor. Łukasz works scientifically in the fields of statistical hypothesis testing and functional data analysis. He also deals with the application of statistical methods and machine learning in practical issues. He has been programming in R for many years and is a co-author of several of its packages available in CRAN and GitHub repositories.
Website: http://ls.home.amu.edu.pl
Introduction to Functional Data Analysis: Modeling, Inference, and Real-Data Applications
Abstract: Functional data analysis (FDA) is a branch of statistics that focuses on modeling and making inferences from observations treated as functions, curves, or surfaces. It provides a powerful approach for analyzing one or more variables measured over time or space, which is a common scenario encountered in various fields. For example, we can consider the measurement of temperature every minute at multiple weather stations over a specific period in a country. Each weather station's temperature values recorded at time points can be modeled as a function, representing a single functional observation. Utilizing a functional representation of time series data helps overcome challenges associated with classical multivariate statistical techniques, such as the curse of dimensionality and missing data. Over the past two decades, a wide range of data analysis methods have been developed specifically for functional data. These methods encompass classification, clustering, dimension reduction, outlier detection, regression, and statistical hypothesis testing. During the presentation, we will explore different aspects and strategies employed in functional data analysis techniques. In particular, we consider the resampling methods for hypothesis testing problems and regression. The presentation will feature real data examples prepared using the R programming language to illustrate the problems and methodologies discussed.
Our Partners
This year's Workshop is offered in cooperation with the Research Center Trustworthy Data Science and Security.