FAIR Workshop on Prediction Modeling
The interdisciplinary research area FAIR organizes a two-day workshop on Prediction Modeling.
In this workshop, participants will be introduced to methods for the prediction of individualized treatment effects and to using (deep) neural networks for statistical modeling.
Our invited speakers are:
- David Rügamer (LMU Munich)
- Thomas Debray (Smart Data Analysis and Statistics)
- Long Nguyen (University of Copenhagen)
When and where
Date and Time: October 11 and 12, 2023. 9:00-13:00 CET
Location: TU Dortmund University, Mathematics Building (Vogelpothsweg 87), room M/E27
Virtual: Online in Zoom (a link will be sent only to registered participants by email)
How to register
Registration is necessary both for on-site and online participation. In order to register for this workshop, please fill out the following online form: link
The deadline for registration is 30th September 2023.
Invited Speakers
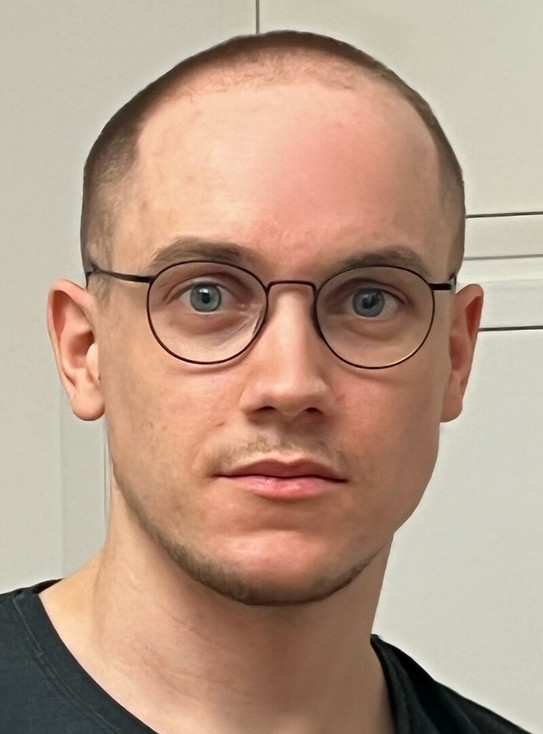
David Rügamer
Prof. Dr. David Rügamer is currently head of the Data Science group at LMU Munich. Before he was interim professor for computational statistics at the RWTH Aachen and TU Dortmund. His research is mainly focused on the intersection of statistical modeling and deep learning.
From Statistical Modeling to Deep Learning
Abstract: Modern data regimes require advanced statistical modeling techniques to allow for big or high-dimensional data, complex data generating processes and non-tabular data modalities such as images or texts. Starting from how to embed classical statistical regression models in a neural network, this workshop will step-by-step explain how to incorporate (deep) neural networks into the statistical analyses. In this context, the course will also cover the basics of neural networks and delve into their implementation and training in TensorFlow and keras using R.
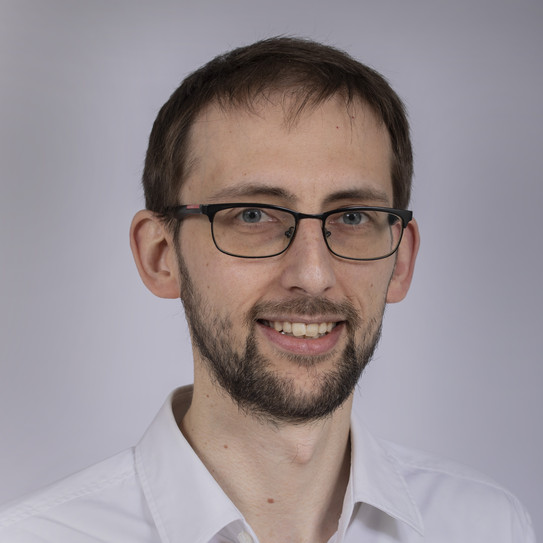
Thomas Debray
Thomas Debray is the founder of Smart Data Analysis and Statistics B.V., a consulting firm that specializes in the development and practical application of innovative statistical methods. During his years of experience as a scientist, he led the development of advanced statistical methods, specifically tailored for risk prediction and meta-analysis.
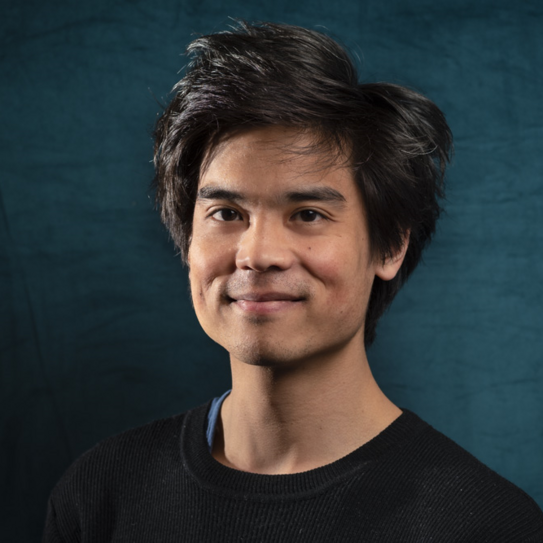
Long Nguyen
Dr. Long Nguyen is currently tenure track assistant professor at the University of Copenhagen. He is conducting epidemiological/statistical methodological research at the nexus of causal inference and prediction modelling with medical and public health applications. Together with Dr. Thomas Debray and Prof. Robert Platt, he is also editor of an upcoming book titled ‘Comparative Effectiveness and Personalized Medicine Research Using Real-World Data’.
Personalized medicine research: an introduction to treatment effect estimation
Personalized medicine is revolutionizing healthcare by tailoring treatment decision- making to patients. This seminar delves into the critical aspects of causal inference and prediction modeling for the advancement of personalized medicine. It will cover the following key points:
- Treatment Effect: Concepts and Definitions (45min)
-
Why Estimate Population Average Treatment Effects (ATE, ATT, ATU)?
We explore the rationale behind estimating population-level treatment effects rather than individual-level effects.
-
Patient A is not Patient B: Conditional Average Treatment Effects (CATE)
We discuss the concept of conditional average treatment effects, emphasizing the heterogeneity of treatment responses across patients.
-
- Heterogeneous Treatment Effects According to Baseline Risk (45min)
-
Development of Risk Prediction Models
We delve into the creation of risk prediction models.
-
Assessment of Risk Prediction Model Performance
We present evaluation metrics like discrimination and calibration of risk prediction models.
-
R Package “metamisc”
An introduction to the R package “metamisc” for implementing these methodologies.
-
- Heterogeneous Treatment Effects According to Baseline Covariates (45min)
-
Development of CATE Prediction Models
We present the development of models that predict CATE to identify subgroups of patients who may benefit most from specific treatments.
-
Assessment of CATE Prediction Models
Similar to risk prediction models, we present the performance metrics of CATE prediction models using discrimination and calibration.
-
R Package “precmed"
An overview of the R package “precmed” for implementing CATE prediction.
-
- What’s next? (20min)
-
Population Average Decision Effects According to Prediction Models
We discuss the importance of estimating the effect of decision-making informed by prediction models.
-
Synthesis of Randomized Studies & Real-World Data
We discuss the synthesis of data from randomized controlled trials and real-world data to advance personalized medicine.
-